Key Insights into Statistical Quantitative Analysis Tools
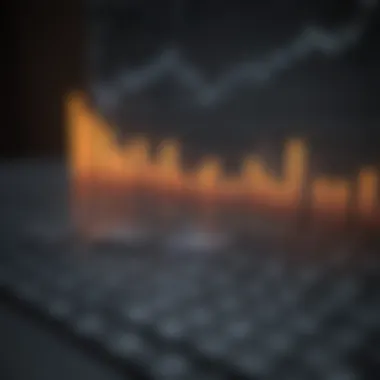
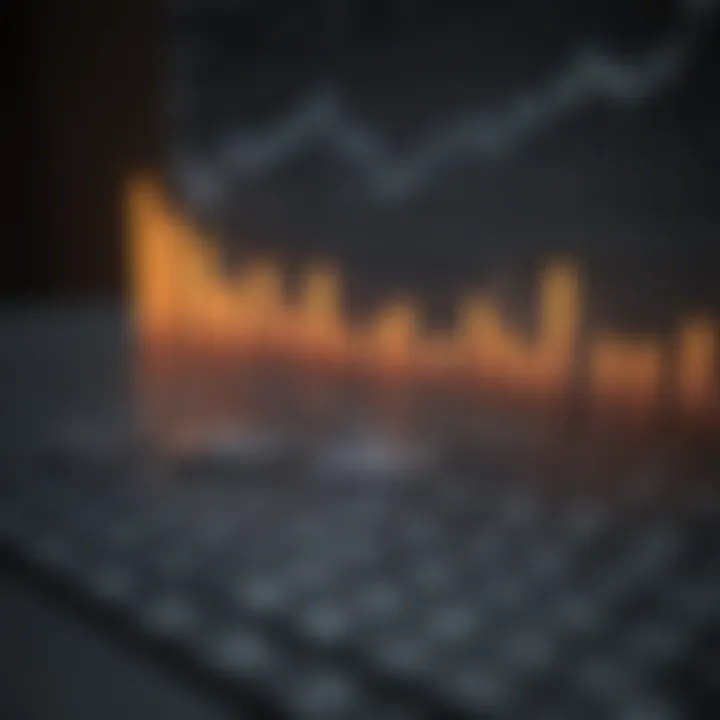
Investment Dictionary
In the world of finance, precise language makes all the difference. This section lays down the foundational terminology that every investor should familiarize themselves with. Understanding these terms can sharpen one’s analytical prowess and inform better financial decisions.
Definition of Key Terms
- Statistical Analysis: This is the process of collecting and scrutinizing every data sample in a set of items from which samples can be drawn. It's the backbone of effective investment decision-making.
- Quantitative Analysis: This refers to the use of mathematical and statistical models to understand behavior and predict outcomes. In finance, it’s vital for pricing strategies and risk management.
- Regression Analysis: A statistical method used to determine the relationship between variables. For instance, it can help investors see how changes in one factor, like interest rates, can impact stock prices.
- Volatility: This term describes the degree of variation in trading prices over time. High volatility can mean greater risk and potential reward; understanding this concept helps in strategizing investments.
Understanding these terms isn't just about rote memorization; they frame how you approach financial analysis.
Explanation of Financial Concepts
Grasping basic financial ideas is crucial for any investor. Here's a brief overview of concepts that regularly pop up in the investment landscape:
- Market Capitalization: The total dollar market value of a company's outstanding shares. It indicates the size of the company and helps in comparing with others in the sector. For example, a company worth over $10 billion is considered a large-cap stock.
- Diversification: A strategy that involves spreading out investments across different assets to lower risk. It's like not putting all your eggs in one basket; you might enjoy the ride if one area hits a bump.
- Asset Allocation: This refers to the decision on how to distribute capital among different investment types – stocks, bonds, real estate. It’s all about striking a balance between risk and reward.
"In investing, what is comfortable is rarely profitable." – Robert Arnott
Arming yourself with these terms and concepts provides the groundwork needed to tackle more advanced strategies and tools of quantitative analysis.
Expert Advice
No matter if you're just starting or have been navigating the choppy waters of investment for years, there's always a lesson to learn. This section distinguishes between advice tailored for beginners and seasoned investors.
Tips for Beginner Investors
- Start Small: Begin with what you can afford to lose. The markets can be unpredictable, and it’s better to learn with smaller sums.
- Educate Yourself: Focus on building your knowledge base. There are numerous free resources like Wikipedia and forums like Reddit to gather insights.
- Set Realistic Goals: Define what you want to achieve with your investments, whether it’s wealth accumulation or saving for retirement, and plan accordingly.
Advanced Strategies for Seasoned Investors
- Utilize Risk Management Tools: Tools like stop-loss orders or options can help mitigate losses in volatile markets. Knowing when to cut your losses can mean the difference between a minor setback and a major hit to your capital.
- Stay Updated on Market Trends: Daily news and analysis can provide context to your investments. Follow credible sources to make informed decisions.
- Experiment with Algorithmic Trading: If you're feeling adventurous, consider strategies that use algorithms for trade execution based on quantitative analysis, sometimes even just a well-designed spreadsheet can enhance this approach.
Through diligent learning and applying these strategies, investors can elevate their game, whether they’re just stepping into this vast arena or already adept at manipulation of the financial tools available.
Preface to Statistical Quantitative Analysis Tools
Statistical quantitative analysis tools are the compass and map in the vast ocean of data. Their relevance can't be overstated, especially for investors who navigate through numbers and trends to make informed decisions. Having a strong grasp of these tools not only helps in deciphering complex data but also in making strategic moves that could be the difference between gain and loss.
Definition and Purpose
Statistical quantitative analysis tools are methodologies and systems used to collect, analyze, and interpret numerical data. Their primary purpose is to transform raw data into actionable insights. To put it simply, they allow investors to see patterns, trends, and correlations that are not immediately obvious. This helps not just in making decisions but also in predicting possible outcomes based on historical data. Software tools, statistical tests, and data visualization techniques venture beyond the surface to provide clarity in quantitative analysis.
Historical Context
The roots of statistical analysis can be traced back centuries. From the early days of census data collection by governments to modern-day algorithms that crunch big data, these tools evolved alongside advancements in mathematics and computing. Historical figures such as Karl Pearson and Ronald Fisher laid the groundwork by developing foundational concepts in statistics. Fast forward to today, and we find ourselves in an age where technology enables us to perform complex analyses in mere seconds. Just imagine how the financial analysts of the past would marvel at today’s capabilities!
Importance in Finance
In finance, the stakes are genuinely high. The correct implementation of statistical quantitative analysis tools can dramatically elevate an investor’s decision-making capability. When conducting analyses like risk assessment or market trend evaluations, these tools turn vague numbers into potent strategies. They facilitate:
- Data-driven decision making: Helps investors avoid basing choices on gut feelings, which can be risky.
- Enhanced forecasting: Offers a glimpse into potential future scenarios, allowing for better preparation.
- Performance measurement: Ensures that ongoing strategies can be evaluated and fine-tuned as necessary.
"In finance, data is not just numbers; it’s the very lifeblood of every strategic decision."
Grasping the essence of statistical quantitative analysis is no longer just an optional skill for finance professionals. As the financial landscape continues to become more complex, being adept at these tools is more essential than ever for both novice and seasoned investors alike.
Types of Statistical Analysis Tools
When diving into the world of statistical quantitative analysis, knowing the different types of analysis tools is crucial. These tools serve as the backbone of data interpretation, helping investors and analysts derive meaning from often complex data sets. Understanding these tools enables one to make informed decisions, formulate strategies, and optimize performance. In the realm of finance, these tools are not just nice to have; they are essential in navigating the intricacies of financial markets.
Descriptive Statistics
Descriptive statistics form the foundation of any statistical analysis. At its core, this type involves summarizing and organizing data to provide a clearer picture of what the numbers are telling us. Common descriptive statistics include measures of central tendency, such as the mean, median, and mode, as well as measures of variability, like range and standard deviation. These metrics give a snapshot of the characteristics of a dataset, allowing investors to understand its general trends and patterns.
For instance, if an investor is looking at the returns of different stocks over the last year, descriptive statistics can highlight which stocks performed well and which didn’t. With tools like SPSS or even Excel, analysis can become more straightforward. This not only aids in performance evaluation but also allows for quick comparisons between different investment opportunities without diving into deeper statistical nitty-gritty.
Inferential Statistics
While descriptive statistics provide snapshots, inferential statistics goes a step further by enabling generalizations to be made about a larger population based on a sample. The essence here lies in hypothesis testing and confidence intervals. This is incredibly useful for investors who want to predict trends based on historical data or sample studies.
Imagine an analyst looking at the performance of a small selection of mutual funds to gauge overall market performance. By applying inferential techniques, they can make educated guesses about how the market may perform based on their findings. Understanding concepts like p-values and confidence levels is vital in this sphere, as they help in assessing the reliability of results derived from these models.
Predictive Analytics
Predictive analytics steps into the arena of forecasting future outcomes based on historical data patterns. This tool harnesses statistical algorithms and machine learning techniques to analyze current and past datasets to make predictions about unseen future events. In finance, this means that data which illustrate past market trends can be used to create models for predicting stock prices, sales figures, or potential risks.
Take the case of regression analysis, a powerful predictive tool that can analyze relationships between variables. If an investor discovers a correlation between economic indicators and stock performance, predictive analytics can fine-tune their investment strategies accordingly. Having software like R programming or Python libraries further enhances this capability, making complex calculations more manageable and accessible.
Multivariate Analysis
When dealing with multiple variables simultaneously, multivariate analysis comes into play. This method allows a more comprehensive examination of the interrelationships between various factors affecting financial outcomes. Investors may find value in understanding how different variables interplay, whether it’s analyzing the impact of interest rates, inflation, and market volatility all at once.
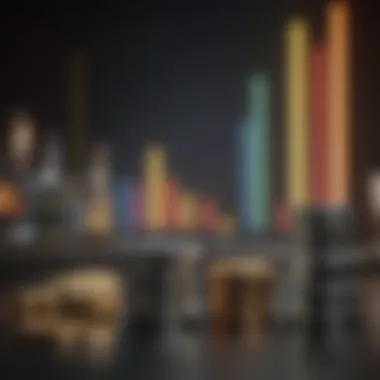
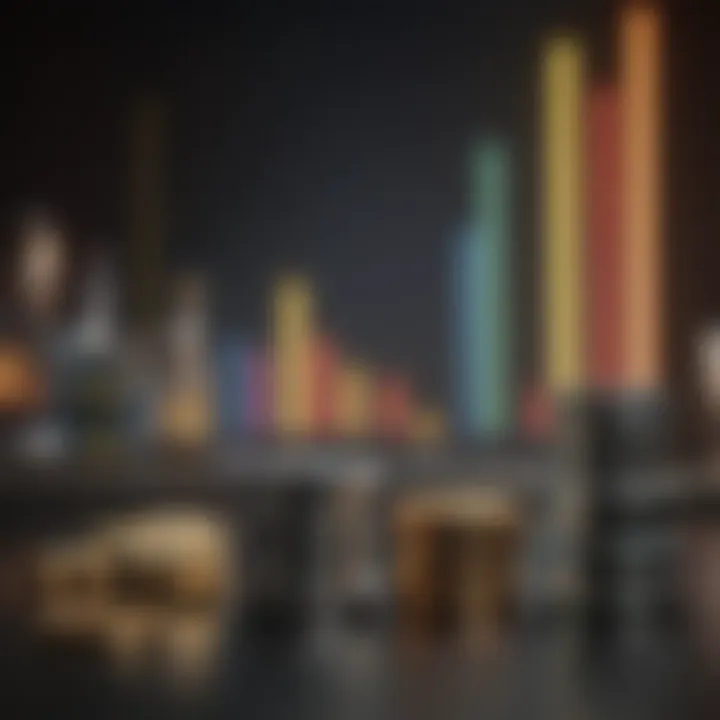
This analysis might seem daunting at first, but tools like Tableau make it significantly more digestible by providing intuitive visual representations. For example, an analyst could visualize how stock prices react under various economic conditions, thus gaining insights that can shape investment decisions.
Key Tools and Software
In the realm of statistical quantitative analysis, selecting the right tools and software is absolutely paramount. The decisions made through data-driven insights significantly influence financial outcomes. With various tools available, understanding their unique features and capabilities can clarify the decision-making process.
Utilizing the appropriate software can enhance efficiency, improve accuracy, and provide deeper insights into market behavior. From statistical analyses to complex visualizations, these tools streamline what might otherwise be a daunting task, allowing investors to focus on strategy and execution.
SPSS
SPSS, short for Statistical Package for the Social Sciences, has carved a niche for itself as a versatile tool. Initially developed for social science research, it has been embraced by finance professionals owing to its user-friendly interface and robust functionalities.
This software excels at handling large datasets, making it a favorable option for financial analysts needing to perform sophisticated analyses without getting bogged down in complexities. Its features include:
- Data management capabilities that facilitate data cleaning and organization.
- A wide range of statistical tests, from t-tests to regression analyses, all accessible through menus.
- Visual output options, allowing users to create clear graphs and charts.
Moreover, SPSS supports scripting through Python, enabling users to customize their analyses further. However, the cost could be a limiting factor for smaller entities.
R Programming
R Programming is a powerful tool that appeals to those who enjoy diving deep into statistical methods. Unlike SPSS, R is an open-source language, which means it is free to use and has a strong community contributing to a vast array of packages tailored for specific analyses.
Some of the strengths of R include:
- Flexibility in conducting a wide range of statistical techniques.
- An extensive collection of libraries like for data visualization and for data manipulation.
While the learning curve may be steeper compared to other software, once mastered, R can unlock advanced analytical techniques that are particularly beneficial for seasoned investors.
Python Libraries
Python has emerged as a formidable player in statistical quantitative analysis, with libraries such as Pandas, NumPy, and SciPy providing a comprehensive toolkit for data manipulation and statistical testing.
Investors often favor Python for its ease of integration with web applications and extensive use in machine learning. Key advantages include:
- Pandas for data manipulation and cleaning.
- SciPy for scientific computing and engineering.
- Matplotlib and Seaborn for extensive visualization options.
Because Python is open-source, a plethora of resources and community support exists, which can prove invaluable for problem-solving or learning new techniques. This accessibility makes it attractive to both beginner and experienced analysts alike.
Excel for Analysis
Excel remains a staple in financial analysis despite the rise of more specialized tools. Its widespread familiarity makes it a go-to for many analysts, providing a straightforward platform for conducting basic statistical analysis.
Some noteworthy points about using Excel include:
- Ease of use: Many people are comfortable with its interface, allowing for rapid data input and manipulation.
- Built-in functions for statistical calculations such as mean, standard deviation, and correlation.
- Success in visualizing data with charts and graphs.
While it may fall short in handling large datasets or conducting advanced analyses compared to R or Python, its practicality for day-to-day tasks should not be underestimated.
Tableau for Visualization
Tableau shines especially when it comes to creating compelling visualizations. This tool focuses primarily on transforming raw data into visually appealing dashboards that can communicate insights effectively.
Key aspects of using Tableau are:
- User-friendly interface that allows for drag-and-drop functionality.
- Ability to connect multiple data sources seamlessly.
- Interactive dashboards making it easier for stakeholders to grasp complex data quickly.
However, Tableau's strength lies in its visual output rather than statistical computations, making it an excellent complement to more analytical tools. The investment can be worthwhile for organizations looking to enhance their presentation of analytical results.
Each tool offers unique benefits, and the choice often depends on the specific requirements and the users’ comfort level with technology.
Core Statistical Concepts
In the world of quantitative analysis, understanding core statistical concepts is paramount. These are the building blocks that form the foundation of data-driven decision-making in finance. They help investors interpret data meaningfully, allowing them to extract insights and make informed choices. Gaining a grasp of these concepts not only demystifies the sea of numbers but also empowers investors to approach financial problems with confidence and clarity.
Population vs Sample
The distinction between a population and a sample is crucial when engaging with statistical analysis.
- Population refers to the entire group that a researcher is interested in. For example, if an investor is analyzing the performance of all tech stocks in the S&P 500, that’s their population.
- Sample is a subset of the population used for statistical analysis. It is often impractical or infeasible to gather data from an entire population. For instance, an investor may choose to analyze 50 tech stocks as a representation of the overall market.
Understanding this difference allows practitioners to assess how representative their sample is of the entire population. If the sample isn't adequately selected, it could lead to skewed insights, which may mislead financial decisions.
"In statistics, the right sample can make a world of difference. A poor choice can throw off results beyond recognition."
Types of Variables
Variables are the different characteristics or attributes that can be measured and analyzed. In the context of financial analysis, variables can be categorized into various types:
- Qualitative Variables: These are non-numeric and usually descriptive, such as categories like 'high-risk' or 'low-risk' stocks.
- Quantitative Variables: These are numeric and can be divided into two subcategories:
- Discrete Variables: These can only take certain specific values, like the number of shares traded.
- Continuous Variables: These can take any value within a range, such as stock price.
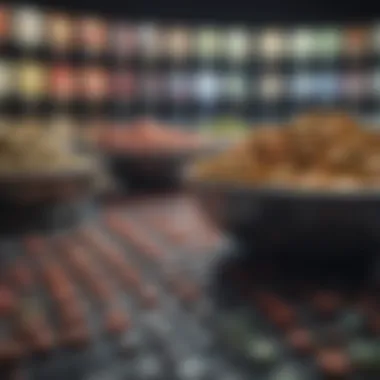
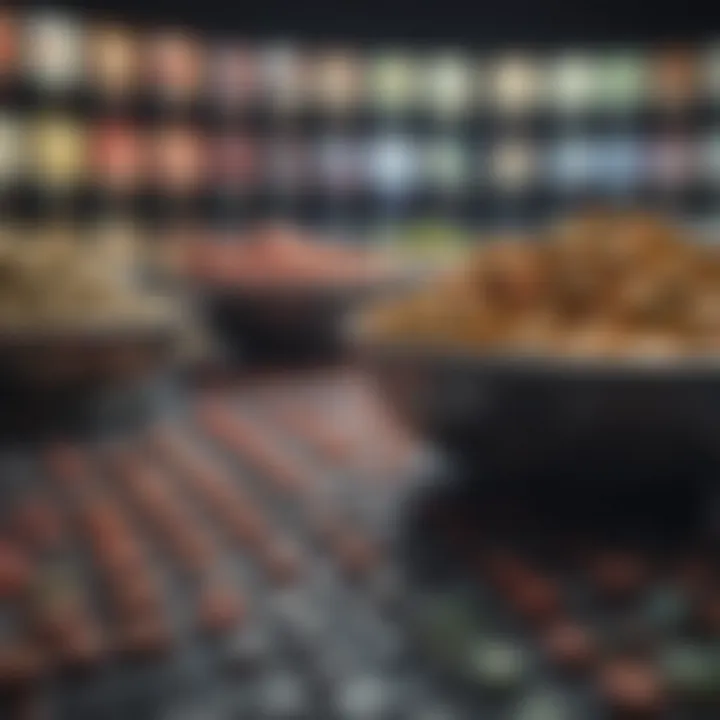
Understanding the types of variables helps determine suitable analysis methods and tools. For example, regression analysis might be used for quantitative variables, while clustering techniques may be more apt for qualitative data.
Hypothesis Testing
Hypothesis testing is a structured process for assessing assumptions about a financial dataset. It involves two competing hypotheses: the null hypothesis, which proposes no effect or difference, and the alternative hypothesis, suggesting otherwise.
Let's say an investor wants to test whether a new investment strategy outperforms a benchmark. The steps usually look like:
- State the hypotheses: For instance, the null hypothesis could state that the strategy does not outperform the benchmark, while the alternative states that it does.
- Select a significance level: Often set at 0.05, this reflects the probability of rejecting the null hypothesis when it is actually true.
- Conduct the test: Use statistical tools to analyze the data and calculate the p-value.
- Draw conclusions: If the p-value is less than the significance level, the null hypothesis is rejected, indicating there is significant evidence against it.
Hypothesis testing is vital for investors looking to validate strategies and make evidence-based decisions.
Confidence Intervals
Understanding confidence intervals (CIs) is central to interpreting the results of statistical analysis. A confidence interval provides a range of values that is likely to contain the population parameter with a certain level of confidence, typically 95%.
For instance, if an investor calculates a 95% confidence interval for the expected return on an investment to be between 6% and 10%, they can be fairly certain that the true return lies within that range.
Knowing how to construct and interpret confidence intervals enables investors to communicate the uncertainty associated with their statistical estimates effectively.
In summary, the core statistical concepts encompassing population versus sample, types of variables, hypothesis testing, and confidence intervals are not only fundamental to statistical analysis but also instrumental in refining decision-making in financial contexts. Emphasizing understanding of these principles facilitates deeper insights into data and fosters more effective investment strategies.
Applications in Financial Analysis
As the dust settles on theoretical frameworks, the real action in finance happens on the ground, where statistical tools play a vital role. Applying quantitative analysis in finance leads to informed decision-making, risk management, and strategy formulation. This section dives into various applications that underline the relevance of statistical quantitative analysis in shaping financial landscapes.
Risk Assessment
In the world of finance, risk is as common as the air we breathe. Risk assessment, grounded in statistical principles, enables investors and analysts to quantify the likelihood of adverse events impacting investments.
- Identifying Risks: Through methods such as Value at Risk (VaR), analysts can estimate potential losses in different market conditions. By examining historical data and market trends, they can identify potential pitfalls before they become crises.
- Measuring Impact: Once risks are identified, it’s essential to gauge their potential impact. Tools like simulation modeling can provide insights into how varied scenarios could affect the portfolio. This prepares investors to brace themselves for the worst.
- Dynamic Adjustments: Good risk management is not static. By regularly updating risk models and adapting to emerging trends, investors can pivot strategies swiftly. This dynamic approach can be a game-changer in volatile markets.
Portfolio Optimization
Imagine crafting a portfolio that not only meets your financial goals but also minimizes risks. That’s the essence of portfolio optimization. Using quantitative methods, investors can mix and match assets to strike the right balance between risk and return.
- Mean-Variance Optimization: Developed by Harry Markowitz, this technique minimizes risk for a given return or maximizes return for a given level of risk. In simpler terms, it’s like finding the sweet spot among various options.
- Asset Allocation Models: Determining how to diversify investments across asset classes is crucial. Statistical tools help analyze correlations between assets to inform allocation decisions. By doing so, investors can avoid overexposure to any single asset class.
- Continuous Reevaluation: Market conditions fluctuate. Ongoing quantitative analysis ensures that portfolios stay aligned with personal goals and market realities. A one-size-fits-all approach is not effective in today’s complex financial environment.
Market Trend Analysis
Understanding market trends is akin to reading the tea leaves of finance. Statistical tools empower analysts to discern patterns within seemingly chaotic data, leading to actionable insights.
- Time-Series Analysis: This technique tracks price movements over time, helping investors spot trends and seasonal patterns. It’s invaluable for making predictions about future behavior based on historic performance.
- Sentiment Analysis: Recent developments involve tracking social media and news sentiment to gauge market emotions. Tools that quantify public sentiment through keywords and phrases can signal potential market movements before they happen.
- Technical Indicators: Various statistical indicators, such as moving averages and Bollinger Bands, assist in illustrating the underlying trends in a stock or market. They help investors determine entry and exit points in trading.
Performance Measurement
Ultimately, if one doesn't measure performance, they’re merely shooting in the dark. Statistical tools offer frameworks for gauging investment effectiveness, providing crucial feedback for future strategies.
- Benchmarking: By comparing the performance of a portfolio against a benchmark index, investors gain perspective. This insight helps in assessing whether their strategies are yielding desired returns relative to the market.
- Attribution Analysis: This allows investors to determine which factors contributed most to portfolio performance. Were returns driven by asset allocation or individual security choices? Statistical methods isolate these effects, refining decision-making.
- Risk-Adjusted Returns: Simply focusing on returns is misleading; it’s important to consider the risks taken to achieve those returns. Ratios like Sharpe and Sortino offer insights into performance relative to risk taken, helping investors make more informed comparisons.
A quantitative approach does not guarantee success, but it significantly raises the odds by minimizing guesswork and grounding decisions in data.
Challenges in Quantitative Analysis
Statistical quantitative analysis is not without its hurdles. Recognizing these challenges is crucial for anyone who aims to navigate the complexities of financial analysis effectively. These challenges can undermine the analysis process, leading to misinformed decisions, wasted resources, or even financial losses. That's why understanding these pitfalls is foundational for both novice and seasoned investors.
Data Quality and Integrity
One of the cornerstones of any statistical analysis is the quality of data. Garbage in, garbage out, as they say. If the data fed into your models is flawed—be it outdated, incomplete, or inaccurate—then the results of your analysis will likely reflect those imperfections.
- Source Reliability: Gathering data from reputable sources is key. Data obtained from questionable sites or small-time surveys can skew results significantly.
- Regular Updates: Markets change rapidly. Using stale data poorly affects analyses. Continuous data updates help maintain integrity.
- Cleaning Data: Raw data often contains errors. Miscalculations, duplicates, or missing values need scrubbing before they can be of any use.
Failing to address data quality can lead to decisions based on false assumptions, like thinking a strategy is sound when it's based on faulty information. This is particularly critical in finance, where a misstep can lead to substantial losses.
Overfitting Models
A common trap in quantitative analysis is overfitting. This happens when a model is too complex and captures noise along with genuine trends. Essentially, it becomes a "jack of all trades, master of none."
- Model Complexity: Using an overly complicated model might seem smarter at first. However, it risks misguiding you about future data behavior.
- Data Regex: Training your model on too much historical data can lead to overfitting, as it learns noise instead of the actual signal that might predict future outcomes.
- Regularization Techniques: Utilizing techniques like Lasso or Ridge regression can help combat overfitting by penalizing excessive model complexity.
Models that are overfitted might perform well on past data but fall flat due to their inability to generalize when new cases are encountered. In a dynamic market, this could translate to lost opportunities.
Misinterpretation of Results
Even with high-quality data and well-fitted models, results can still be misunderstood. Misinterpretations can cloud judgment.
- Misleading Averages: Simply relying on mean values can obscure the entire story. For instance, a few large outliers can skew these averages, making a situation appear more favorable than it is.
- Causal vs Correlation: Just because two trends move together doesn't mean one causes the other. A classic tongue-twister in statistics, this misunderstanding often leads to poor strategic decisions in finance.
- Visualization Pitfalls: Bad graphs can tell a misleading story. Ensure your visual tools accurately represent the data to prevent misinterpretations.
In the world of finance, interpreting the numbers without missteps can either make or break your analysis.
In summary, while quantitative analysis tools offer powerful insights, they are not foolproof. Challenges such as data quality, model complexity, and potential misinterpretation must be navigated with caution and diligence. Understanding these issues is essential for making sound financial decisions.
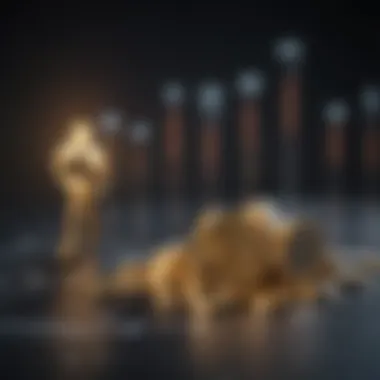
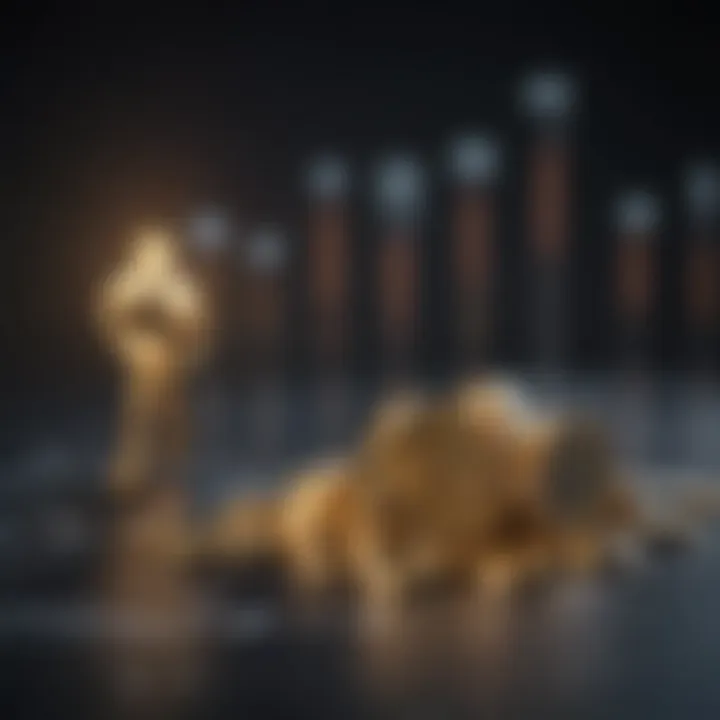
Selecting the Right Tool for Your Needs
Choosing the right tool for statistical quantitative analysis is no small feat. As the landscape of financial tools continues to evolve, having a clear grasp of what's available is crucial for making the right decisions. Whether it's for crunching numbers, visualizing data, or forecasting trends, the tools you select play a pivotal role in shaping your analytical prowess and investment strategies.
Understanding Your Objectives
Before diving into the myriad of options out there, it’s essential to pin down your main objectives. Why are you conducting this analysis? Is it for risky endeavors, or are you focusing on long-term investments? Perhaps you seek to optimize your portfolio or maybe it's about assessing market trends. Clarifying your goals helps in sifting through the clutter and zeroing in on software that aligns with your needs.
- If you're into real-time investment tracking, using tools that offer up-to-the-minute data can be paramount.
- For longitudinal studies, software that can handle historical data trends likely suits you best.
Understanding what you want out of your analysis will guide your decision like a beacon on a foggy night.
Evaluating Software Features
Once you've set your sights on your goals, examining the features of various tools is the next logical step. Not all software is created equal. Here’s what to keep an eye out for:
- User Interface: Ensure it’s user-friendly. A complicated interface can waste your time.
- Statistical Techniques: Does the tool offer the statistical methods you need? Different software varies in techniques offered.
- Visualization Options: A robust visualization feature allows you to make sense of numbers quickly.
- Data Import Options: Can it easily pull data from different sources? This is especially important if you regularly work with varied data sets.
Discovering these features can either bolster your capabilities or create unnecessary hurdles. It all boils down to how well the tool serves your specific functions.
Integration with Other Systems
Lastly, your chosen analysis tool should not exist in a vacuum. In today’s interconnected world, how well a tool integrates with existing systems can either enhance your workflow or throw a monkey wrench in the gears.
Consider the following:
- Financial Platforms: Does it sync smoothly with platforms like Bloomberg or Yahoo Finance?
- Data Storage: Can it link with databases like Oracle or SQL servers?
- Collaboration Tools: If you're working on a team, interoperability with systems like Slack or Asana can help streamline communication.
Ensure your choices create a cohesive environment. The last thing you want is a great tool that works in isolation, effectively becoming an anchor instead of a sail.
When in doubt, reach out to communities on platforms like Reddit to gather insights from users on their experiences with different tools.
In summary, the path to selecting the right statistical analysis tool requires a good deal of introspection, research, and due diligence. Your choice should align not merely with your current needs but should also anticipate your future analytical endeavors.
Future Trends in Quantitative Analysis
In today’s financial landscape, the tools used for quantitative analysis are rapidly evolving. The significance of understanding future trends can't be overstated. Emerging technologies and methodologies are shaping how investors approach data analysis. Keeping an eye on these developments is crucial for anyone looking to navigate the complex world of finance successfully.
Big Data and Machine Learning
The explosion of Big Data has fundamentally altered the playing field for quantitative analysis. These vast datasets, much larger than traditional models can handle, provide a treasure trove of information. Machine learning algorithms come into play here, sifting through immense volumes of data, identifying patterns and making predictions that are often beyond human capacity. This means that investment decisions can be made with greater accuracy, taking into account numerous factors that were previously unconsidered.
- Advantages of Integrating Big Data:
- Enhanced predictive capabilities
- Improved risk assessment
- Faster analysis through automated processing
By employing machine learning techniques, investors can adjust their strategies in real-time, responding to market changes almost instantaneously. For instance, imagine a hedge fund applying machine learning models that analyze news sentiment and market trends simultaneously. These insights can provide an edge that traditional methods simply cannot match.
Real-Time Analysis
In the world of finance, timing is often everything. Real-time analysis is becoming a non-negotiable requirement for savvy investors. The ability to monitor markets, news releases, and other relevant data as it happens allows traders to react promptly to emerging opportunities or threats.
Having access to tools that provide real-time insights can transform the decision-making process significantly. A few things to keep in mind about real-time analysis include:
- Immediate Data Access: Time delays can be costly, hence why tools that deliver live updates are invaluable.
- Adaptive Strategies: Rapid access to fresh information enables investors to pivot their strategies quickly based on newly available data.
Investors harnessing such capabilities can not only ensure they are reacting to the market but also proactively positioning themselves where potential gains are greatest.
Enhanced Visualization Techniques
The way data is presented can have a profound impact on its interpretation. Enhanced visualization techniques are gaining traction, reshaping how quantitative analysis is understood and practiced. These techniques don’t just present numbers; they tell a story, allowing investors to grasp insights at a glance.
- Interactive Dashboards:
Using platforms like Tableau, investors can create dynamic visualizations that update in real-time, making complex data sets more digestible. - Infographic-style Reports:
Reports that use rich visuals engage users more effectively than pages of numbers. This can lead to quicker, more informed decisions.
Investors who can leverage these advanced visualization tools can distinguish themselves in their analysis, transforming raw data into actionable insights.
"In a world awash with data, it's not just what you have but how you present it that makes a difference."
Closure
In wrapping up this exploration of statistical quantitative analysis tools, it’s vital to discern the crux of their importance in today’s financial landscape. The tools and methodologies articulated throughout the article serve as indispensable allies for both amateur and seasoned investors. With an array of analytical methods at one’s fingertips, the potential for informed decision-making discernibly expands. Financial professionals who leverage these tools often find themselves not only enhancing their analytical prowess but also making calculated decisions that can lead to substantial gains.
Here are some key points underscoring the value of these tools:
- Foundation of Analysis: Many investment strategies hinge on the solid grounding offered by descriptive statistics and predictive analytics. Without this bedrock, decision-making can become erratic.
- Risk Management: Utilizing tools such as inferential statistics helps to gauge potential risks associated with investments, equipping investors with the knowledge to navigate uncertainty with confidence.
- Data Interpretation: The nuances of interpreting data via multivariate analysis are crucial. Investors who can dissect and understand the relationships between multiple variables are often the ones who stay ahead in competitive markets.
Moreover, as we plunge deeper into an era characterized by overflowing data streams, the tools for statistical analysis become not just beneficial but essential. The ability to ascertain trends and derive insights from vast datasets is an increasingly sought-after skill. With this, the advantages are clear: those who adapt and adopt these analytical frameworks will likely yield better outcomes in their financial endeavors.
"In the world of finance, not having the right tools is akin to trying to build a house with a hammer in a world that has already embraced power tools."
Summarizing Key Points
Reflecting on the essential elements discussed, it becomes clear that statistical quantitative analysis tools are the keys to unlocking better financial outcomes. From understanding population versus sample dynamics to employing predictive models, the concepts laid out serve as a foundational toolkit. This understanding is supplemented by knowledge of software tools like SPSS and R programming. Each section detailed provides a stepping stone—whether one is investigating portfolio optimization or assessing market risks, the critical takeaway lies in leveraging data to inform decisions.
Encouragement for Further Learning
As you stand at the precipice of financial decision-making, remember that continuous learning is the astonishingly powerful tool that can enhance your investment acumen. Whether you choose to delve into advanced statistical software or engage with online courses focusing on quantitative analysis, the opportunities are boundless.
Moreover, engaging with communities—like those on Reddit or financial forums—can continue to broaden your perspective and uncover the most practical applications of these tools. Staying informed with the latest trends and analyses can separate the great investors from the good ones. Take that leap today, and immerse yourself in the wealth of knowledge that awaits!