Understanding Big Data Business Models for Success
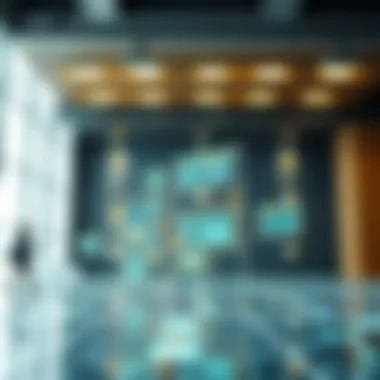
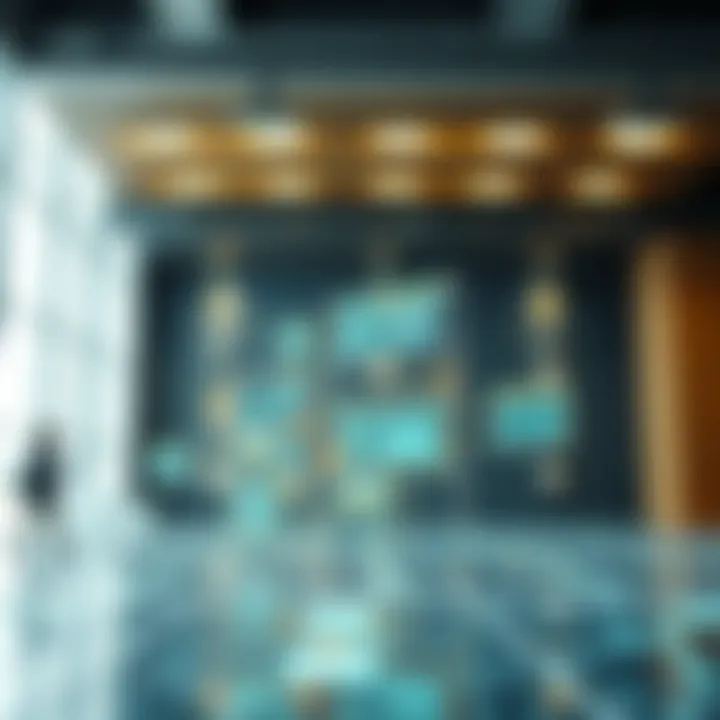
Investment Dictionary
Definition of Key Terms
In the world of big data, there are terms that pop up regularly and might seem intimidating at first. Understanding these terms is crucial for anyone looking to navigate the landscape of big data business models. Here’s a breakdown of some key terms:
- Big Data: Refers to vast volumes of data that are too large or complex for traditional data processing applications. Think of it as a flood of information that can provide valuable insights when properly analyzed.
- Data Analytics: This is the science of analyzing raw data to make conclusions about that information. Essentially, it’s about turning messy data into actionable strategies.
- Monetization Strategies: Techniques employed by companies to generate revenue from data. Different approaches can range from direct sales of raw data to offering analytics tools or insights.
- Data Monetization: The process of generating measurable economic benefits through the sale or use of data.
Explanation of Financial Concepts
Understanding financial concepts related to big data can shape how investors and businesses strategize. Here are some worth noting:
- Return on Investment (ROI): Measures the profit or loss generated in relation to the amount of money invested. In the context of big data, measuring the ROI might include analyzing the savings made by improved operational efficiencies obtained through data insights.
- Cost-Benefit Analysis: This involves comparing the costs of big data initiatives to the benefits derived from them. Businesses need to ascertain whether their investment in data technologies will yield sufficient benefits to justify the expense.
- Market Assessment: Evaluating the potential market demand for data-driven products can guide strategic decisions. Awareness of both competitors and market needs is essential for success.
Expert Advice
Tips for Beginner Investors
- Start with Education: Familiarize yourself with key concepts in big data and its applications. Websites like Khan Academy and Coursera provide valuable resources.
- Follow Industry Trends: Keeping an eye on reports and news in the field of big data can offer insights into burgeoning opportunities. Sources such as Statista can provide essential data and forecasts.
- Engage in Networking: Attend meetups and conferences to connect with industry professionals. Platforms like Meetup can help you find relevant events.
Advanced Strategies for Seasoned Investors
- Diversify Your Portfolio: Engage in a mix of direct investments in data companies, alongside stocks from industries that utilize big data prominently.
- Leverage Artificial Intelligence: Invest in AI analytics tools that can glean insights from vast data pools. Companies such as Microsoft and IBM are leaders in this segment.
- Incorporate Real-Time Analytics: Strategies utilizing real-time data can enhance decision-making processes drastically. Explore businesses that are innovating in this area, like Palantir.
"The only thing worse than being blind is having sight but no vision." - Helen Keller.
This reminds us to look beyond the obvious in the data realm. It's not just about numbers, but the stories they tell.
Preamble to Big Data Business Models
In the contemporary business landscape, the term "big data" has become almost ubiquitous. It's no longer just a buzzword; organizations are now keenly aware of its potential to unlock new avenues for revenue generation and operational efficiencies. Big data has reshaped how companies comprehend their ecosystems, customers, and even their competitors. Gaining a solid foothold in big data business models is essential because these frameworks provide the necessary structure to utilize vast amounts of information effectively.
Leveraging big data allows firms to spot trends and patterns that can inform decision-making, streamline processes, and even predict future outcomes. This isn't just pie-in-the-sky fluff; data-driven insights can lead to tangible improvements and cost savings. A bakery, for example, might analyze purchasing trends to adjust its inventory accordingly, minimizing waste and maximizing sales.
The exploration of big data business models serves several important purposes:
- Understanding Complexity: Big data is intricate and often overwhelming. Dissecting business models helps to unravel its complexities and showcases various strategies that can be employed.
- Identifying Opportunities: Different sectors can harness big data in unique ways. By examining existing frameworks, businesses can identify opportunities tailored to their needs.
- Navigating Challenges: The path isn’t without obstacles. By diving into the intricacies of big data, businesses can better prepare for challenges like data security and ethical considerations.
At the heart of this exploration is the intersection of technology and commerce. Companies that can adeptly navigate this space stand to gain a significant advantage over their competition. It's crucial for not just techies and analysts, but also executives and investors to grasp what big data business models really entail. Knowing what works—and what doesn’t—can transform intuitive decision-making into fact-based strategy.
As we move deeper into the various elements of big data, we will delve into specific definitions and broader business models, setting the groundwork for understanding their implications and applications.
The Role of Big Data in Modern Enterprises
In today’s fast-paced business landscape, the role of big data cannot be overstated. Organizations across various sectors are increasingly relying on data-driven strategies to inform their decisions, improve efficiency, and drive growth. The sheer volume of data generated every day presents a monumental opportunity for enterprises that seek a competitive edge. Without a doubt, harnessing the power of big data can help businesses make informed decisions and optimize operations.
Enhancing Decision-Making Processes
One of the most notable benefits of big data is its ability to enhance decision-making processes. Traditionally, companies relied on intuition, experience, and gut feeling when making critical choices. However, with the advent of big data analytics, organizations can now move towards a more quantitative approach.
- Data-Driven Insights: By analyzing customer behavior, preferences, and trends, businesses can glean insights that were previously hidden. Companies like Netflix utilize algorithms to analyze viewer habits, which allows them to produce targeted content that resonates with their audience.
- Predictive Analytics: Leveraging historical data allows organizations to forecast future patterns. For example, retailers can predict seasonal demand by analyzing past sales data, ensuring they optimize their inventory levels just in time for peak shopping seasons.
- Real-Time Decision Making: The ability to analyze data in real-time gives businesses an edge in rapidly changing markets. Companies such as Amazon can provide tailored recommendations to users based on their browsing history, leading to increased sales and customer satisfaction.
"Big data analytics allows organizations to not just react to trends, but to anticipate consumer needs and market shifts."
Operational Efficiency through Analytics
Operational efficiency is another key area where big data shines. By utilizing analytics, businesses can streamline processes, reduce costs, and enhance productivity.
- Process Optimization: Organizations can identify bottlenecks in their operations by analyzing workflow data. For instance, manufacturers can monitor production processes electronically to minimize downtime and maximize output.
- Cost Management: Big data helps in pinpointing areas where expenses can be cut without sacrificing quality. For instance, airlines use data to optimize flight routes and schedules, directly affecting fuel efficiency and operational costs.
- Performance Tracking: Companies can track the performance of their employees and departments in real-time. This enables managers to make adjustments on the fly, ensuring that teams stay aligned with overall business objectives.
The role of big data is multifaceted, encompassing a range of applications that are essential for modern enterprises. As companies strive to remain competitive, understanding and leveraging big data becomes not just a choice, but a necessity.
Frameworks of Big Data Business Models
The concept of frameworks surrounding big data business models is integral to understanding how organizations can effectively utilize vast amounts of data. These frameworks serve as structural blueprints that guide enterprises in the creation, implementation, and refinement of their data strategies. By focusing on systematic approaches, businesses can harness data not just for collection, but for transformative decision-making. In a world overflowing with information, having a well-defined framework helps to cut through the noise and prioritize what truly matters.
Data-Driven Decision-Making Models
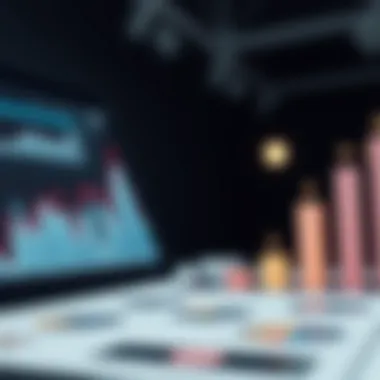
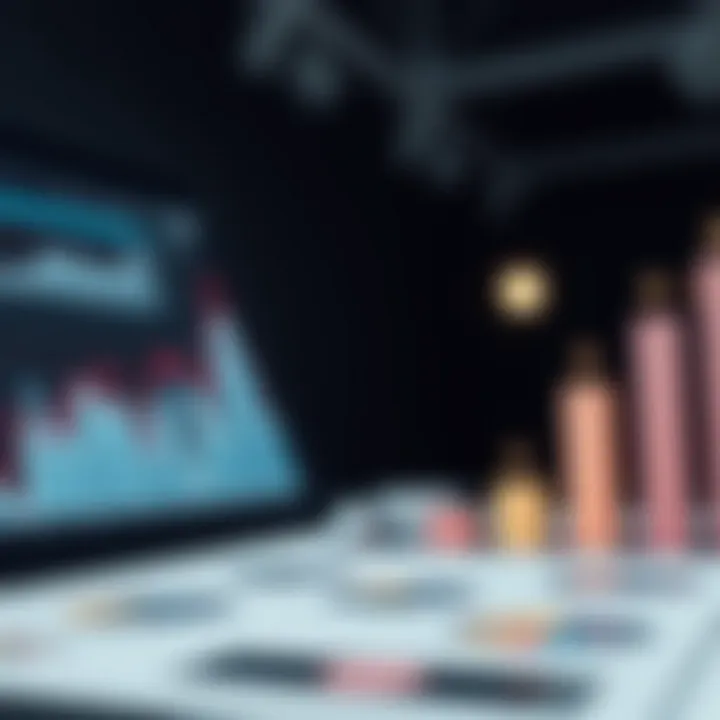
Data-driven decision-making models are all about leveraging data insights to fuel corporate strategies. This isn’t just a fad; it’s become the bedrock of modern business operation. Organizations utilize analytics tools to sift through massive datasets, seeking trends, patterns, and anomalies that may go unnoticed otherwise. When decisions are made based on empirical evidence rather than gut feelings, businesses often see improved outcomes.
- Collecting actionable insights: Companies need to identify which metrics matter most and how to collect data efficiently. This means integrating tools that can easily compile and analyze these metrics.
- Real-time analytics: In the whirlwind of today’s marketplaces, the capacity to respond to data in real-time can set a company apart. Platforms that allow for immediate analysis can foster not just speed but also responsiveness.
Data success stories can be found everywhere. For instance, Netflix utilizes viewing statistics to guide content decisions, ensuring they provide shows that genuinely resonate with viewers. In essence, data-driven decision-making models translate raw data into strategic advantages.
Customer-Centric Models
In an era where consumer preferences constantly shift, customer-centric models act as the compass for navigating these changes. By harnessing big data, companies can gain profound insights into customer desires and behavior patterns. This knowledge can be utilized to tailor offerings that align closely with market demand.
- Personalized marketing techniques: Data allows businesses to customize communications, suggesting products based on past purchases or browsing history. This approach not only enhances customer satisfaction but also increases conversion rates.
- Engaging user feedback: Brands are increasingly relying on customer feedback captured through various channels—surveys, social media interactions, and website analytics. Time invested in understanding these insights pays dividends in product development and customer service.
For example, Amazon's recommendation engine uses customer data to suggest additional items during checkout. This creates a superior shopping experience, enhancing loyalty and driving sales.
Market Research and Analysis Models
Market research models that utilize big data allow companies to broaden their understanding of market dynamics. Rather than singular or periodic research, ongoing analysis helps organizations stay ahead of competitive trends and shifting consumer behaviors.
- Geographic and demographic insights: By capturing data specific to geographic locations and demographics, businesses can better identify target audiences and explore untapped markets.
- Competitive analysis: Big data tools enable firms to analyze competitors’ strategies, revealing their strengths and weaknesses. This intelligence can inform strategic planning and positioning.
As an example, many fintech startups are using big data to customize their services based on regional financial behaviors or preferences. This localized insight can significantly impact service design and offered financial products.
"Big data is not just about numbers; it’s about understanding and translating those numbers into narratives that can shape the future of businesses."
By investing in frameworks that promote effective data utilization, companies can build better paths for innovation, develop enhanced customer relationships, and perform continuous improvements. Thus, understanding and implementing these models isn’t just a nice-to-have, it’s critical for remaining competitive in today’s data-driven environment.
Monetization Strategies for Big Data
Monetizing big data involves more than just collecting information; it's about transforming that data into actionable insights that drive revenue. As businesses recognize the value of data, developing effective monetization strategies becomes crucial. By understanding these strategies, organizations can capitalize on their data assets, improve customer experience, and achieve a competitive edge in the market.
Data Selling and Sharing
In the realm of big data, selling and sharing information can be quite lucrative. However, this strategy hinges on the careful handling of data privacy. Companies can monetize their data by selling access to datasets to third parties, such as advertisers or researchers. Oftentimes, these datasets contain valuable insights that are aggregated and anonymized, minimizing the risks related to data privacy.
"Data is the new oil. It's valuable, but if unrefined, it cannot really be used."
Benefits of Data Selling:
- Revenue Generation: Organizations can generate significant income through data exchanges.
- Market Expansion: By sharing data, companies might tap into new markets or customer segments.
- Networking Opportunities: Collaboration with other firms can lead to partnerships and further innovations.
Still, it's vital to be wary of the ethical considerations surrounding data sharing. Companies must secure informed consent from consumers before distribution and ensure compliance with regulations like GDPR (General Data Protection Regulation) and CCPA (California Consumer Privacy Act). Navigating these waters can be complex, but when done right, the returns can be worthwhile.
Creating Subscription-Based Models
Subscription models represent another compelling strategy for monetizing big data. Companies can offer insights, analytics, or access to platforms on a recurring payment basis. This steady stream of income can foster predictability and stability in business revenue.
For instance, data analytics platforms like Tableau empower organizations to access rich analytics on a subscription basis. Users can rely on ongoing updates and insights tailored to their operations, ultimately improving decision-making abilities.
Key Elements to Consider:
- User Engagement: Continually updating and enhancing the service can retain subscribers.
- Value Proposition: Clearly articulate the benefits subscribers gain.
- Customer Support: Excellent support can improve user satisfaction and retention rates.
However, creating a successful subscription model requires a fine balance; it must provide enough value to users while also considering the cost of acquiring and maintaining that data. It’s essential to keep the offerings fresh and relevant to prevent subscriber churn.
Advertising and Marketing Applications
Big data plays an essential role in modern advertising and marketing strategies. By analyzing consumer data, companies can target their campaigns more effectively, tailoring messages that resonate with specific market segments. This targeted approach not only enhances customer engagement but also results in higher conversion rates.
Using platforms like Google Ads or Facebook Ads, brands can leverage big data for hyper-targeted advertising. These platforms analyze user behavior, preferences, and demographics to serve personalized ads at the right time, ultimately leading to increased return on ad spend (ROAS).
Considerations in Leveraging Big Data for Advertising:
- Data Validation: Ensure the quality of the data being used to avoid misleading insights.
- Privacy Concerns: Adhere to regulations and ethical guidelines to protect consumer data.
- Dynamic Strategy Adaptation: Be prepared to pivot based on analytics and market changes.
With technology continually evolving, understanding how to utilize big data in this context is paramount. The ability to adapt and fine-tune strategies based on data-driven insights can set an organization apart from the pack.
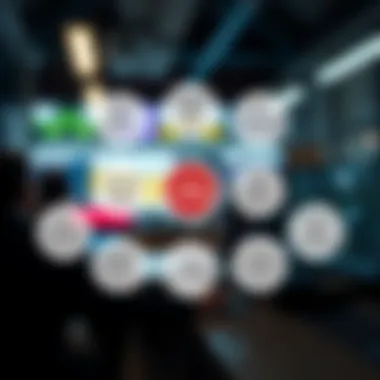
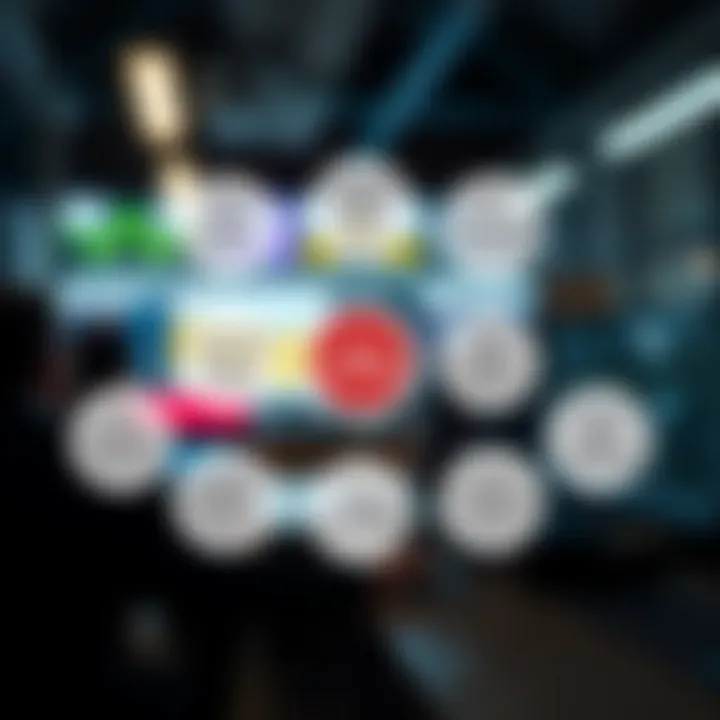
In summary, monetizing big data involves a multifaceted approach, from selling data to creating subscription services to refining advertising efforts. Each strategy offers unique advantages but demands careful consideration of ethical implications and market dynamics.
Technological Infrastructure for Big Data Business Models
In today’s data-driven world, understanding the technological infrastructure behind big data business models is not just beneficial; it's essential. This infrastructure serves as the backbone that supports data collection, storage, analysis, and dissemination. Without a robust technological framework, businesses struggle to harness the insights that big data can provide, leading to missed opportunities and subpar decision-making. The following sections explore the critical components of this infrastructure, offering insights into how they interconnect to empower businesses.
Cloud Computing Solutions
Cloud computing has dramatically transformed the landscape of big data. Companies are moving away from traditional on-premises setups toward scalable cloud solutions. The flexibility of cloud computing allows for the storage of massive amounts of data without the hefty investment in hardware. One significant advantage of cloud services like Amazon Web Services or Microsoft Azure is their ability to scale resources in real time, aligning perfectly with the variable nature of data workloads.
- Cost-Efficiency: Companies only pay for what they use. This model gives businesses the freedom to experiment without risking financial ruin.
- Accessibility: Cloud solutions enable access from anywhere, allowing teams to collaborate effectively, whether in the office or remote.
- Security Features: Leading cloud providers invest heavily in security measures, ensuring that sensitive data remains protected against breaches.
This transition to the cloud is not without its challenges, but the potential for innovation and efficiency far outweighs the drawbacks. Launching a big data initiative can feel like running a marathon, but with cloud computing, the approach turns manageable.
Data Warehousing and Management Systems
Data warehousing plays a pivotal role in big data business models, acting as the organized repository where different data sources converged. Storage solutions like Snowflake or Google BigQuery allow companies to streamline their data management and retrieval processes.
- Centralized Data Access: This keeps all data in one location, making it easier for analytical tools to retrieve and process information.
- Structured Query Language (SQL): Many data warehousing solutions utilize SQL for data manipulation, which is both powerful and user-friendly, catering to both seasoned database administrators and novices alike.
- Real-Time Updates: Advanced data warehouses facilitate real-time data processing. This capability is key for businesses needing immediate insights for swift decision-making.
In a nutshell, an efficient data warehousing system can significantly influence a company's ability to leverage its data assets effectively, turning raw data into valuable insights, seemingly overnight.
Analytical Tools and Platforms
Choosing the right analytical tools is crucial for deriving actionable insights from big data. Today, an abundance of options is available, ranging from traditional business intelligence tools like Tableau to more advanced machine learning platforms like O.ai. Each tool may cater to different business needs, but they collectively serve a common goal: tapping into the vast ocean of data to identify patterns and predict future trends.
- User-Friendly Interfaces: Many analytical platforms are designed with the end-user in mind, providing dashboards that simplify complex data visualizations and decision-making processes.
- Integration with Existing Systems: Most tools offer compatibility with popular data warehousing solutions and cloud platforms, facilitating smooth operations during implementation.
- Adaptability: As analytical needs evolve, these tools can adapt, enabling businesses to stay ahead in a rapidly changing environment.
"In the jungle of big data, the right analytical tools can help you find the gems hidden within the noise."
The thoughtful selection of analytical tools ensures that businesses can adapt to marketplace changes quickly, increasing resilience and competitiveness.
Challenges in Implementing Big Data Business Models
In the realm of big data, navigating through the maze of implementation challenges is no small feat. Organizations must reckon with a host of issues that, if left unaddressed, could derail even the most sophisticated data strategy. The significance of understanding these challenges cannot be overstated, as the success or failure of a big data initiative often hinges on how well organizations prepare for and respond to these complexities. Companies of all sizes should comprehend the multifaceted nature of these challenges not just to avoid pitfalls but to seize opportunities that allied data strategy can offer.
Data Security and Privacy Concerns
With great data comes great responsibility. This adage holds particularly true when it comes to data security. As organizations begin to gather and analyze vast swathes of information, they inevitably become prime targets for cyber threats. The breaches and leaks of sensitive information are not just tough on the pocket; they can tarnish a brand's reputation and erode customer trust. Adopting robust security measures is essential, but it need not signify a leap into an abyss of complexity and cost.
- Balancing Act: Security measures must be balanced with usability. Tight security often means extra steps for users, which might affect productivity.
- Regulatory Compliance: Navigating the regulatory landscape is yet another hurdle. With rules like GDPR setting the bar high, businesses need to continually assess compliance to avoid hefty fines.
- Educating Employees: Training staff to recognize security risks is imperative. Human error often serves as the main gateway for security breaches.
“It’s not just good enough to keep data secure; you have to keep it on its toes.”
Implementing strong encryption methods, regular audits, and establishing clear data governance policies can fortify defenses against data breaches while also respecting user privacy.
Integration with Legacy Systems
Many companies find themselves wrestling with legacy systems that are the backbone of their operations but are often incompatible with modern big data solutions. The challenge is akin to fitting a square peg in a round hole; it might take some engineering but can be done with the right approach.
- Compatibility Issues: New big data tools may not easily integrate with older systems, requiring additional middleware or a complete overhaul of existing IT structures.
- Cost Implications: Upgrading or replacing older systems can be a monumental task, both financially and logistically, often leading to significant downtime if not managed properly.
- Cultural Resistance: Employees accustomed to legacy systems may resist change, seeing new technologies as a threat rather than an opportunity for improvement.
Navigating this integration requires a thoughtful strategy. Often, a staged approach works best. Incrementally rolling out changes allows for smoother transitions and more extensive troubleshooting. Additionally, drawing from examples such as companies like Ford, which confronted its legacy systems head-on, can provide valuable insights on successful adaptation through digital transformation frameworks.
Talent Acquisition and Retention
The war for talent in the big data space is heating up. Organizations are not just looking for data analysts; they are seeking a blend of skills that include proficiency in statistical analysis, programming, and strategic thinking. The complexity of hiring the right talent can often complicate implementation efforts.
- Skill Gaps: Many organizations struggle to find candidates with the right mix of data-centric skill sets combined with business acumen. Many positions remain unfilled due to a shortage of qualified candidates in the job market.
- Retention Strategies: Holding onto skilled employees poses its own challenges. The competitive nature of the job market means that top talent often receives multiple offers, making it essential for businesses to create engaging and growth-oriented environments.
- Investing in Development: Offering continuous learning opportunities is not just nice to have; it’s a necessity. Skills in AI and machine learning are evolving, and training programs can facilitate retention and growth.
As businesses refine their big data strategies, the emphasis on fostering a positive workplace culture that values skilled employees is paramount. Notable companies such as Google and Amazon invest heavily in workforce development, resulting in a dedicated and capable workforce.
Future Trends in Big Data Analytics
The landscape of big data analytics is no static scene; it evolves rapidly in response to technological advancements and user needs. This section is pivotal as it highlights crucial trends shaping the industry. As businesses strive to leverage data for competitive advantage, understanding these trends is essential for making informed decisions. Not only do they unveil new opportunities, but they also pose unique challenges that organizations must navigate. This is particularly relevant for investors and stakeholders keen on optimizing their strategies in an ever-changing market.
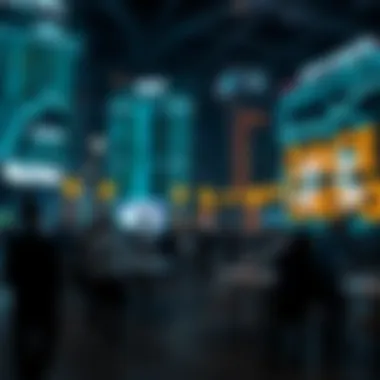
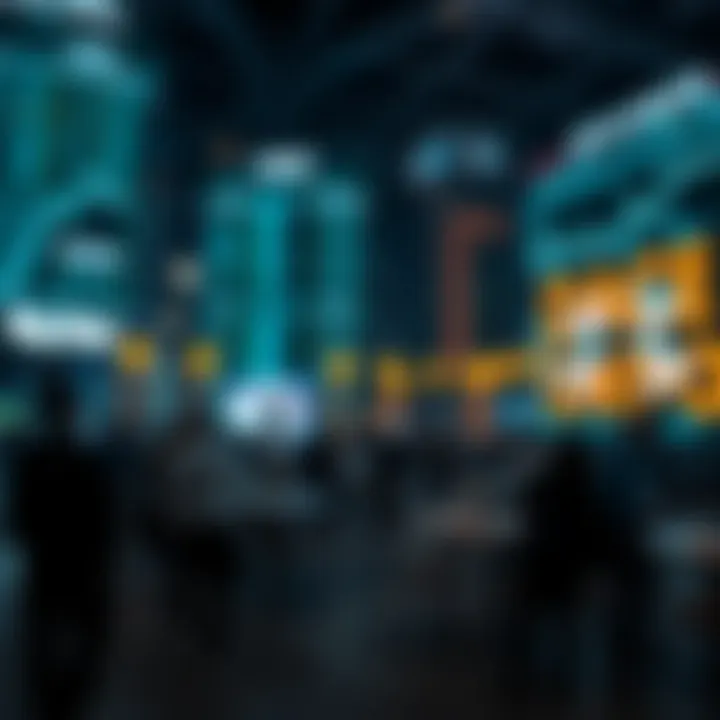
Artificial Intelligence and Machine Learning Integration
The integration of AI and machine learning into big data analytics is akin to adding rocket fuel to a fire. These technologies serve as powerful instruments that can analyze vast amounts of data more efficiently than traditional methods. By automating data processing and analysis, companies can derive insights much quicker, allowing for timely and informed decision-making. This can lead to various benefits:
- Personalization: Businesses can tailor products and services based on real-time customer data, which enhances user experience.
- Predictive Analytics: Firms can forecast trends and behaviors, enabling proactive measures rather than reactive responses.
- Cost Efficiency: Automated processes minimize human error and reduce operational costs over time.
However, investing in AI and machine learning also requires careful consideration. Organizations need to assess whether they have the necessary infrastructure and talent to manage these technologies. Additionally, ethical implications, such as data bias and transparency, must be at the forefront of implementation discussions.
Real-Time Data Processing Advances
The demand for real-time data processing has surged, driven by the need for instantaneous insights. With the proliferation of Internet of Things (IoT) devices, companies are inundated with data that necessitates immediate analysis. In this fast-paced environment, the ability to process and respond to data live can make or break a brand.
Advantages of real-time data processing include:
- Quick Decision Making: Companies can react promptly to market changes or customer feedback, ensuring relevance.
- Enhanced Operational Efficiency: By immediately processing data, businesses can streamline operations and identify areas for improvement.
- Competitive Edge: Organizations that leverage real-time insights can stay a step ahead of their competitors.
As firms adopt these technologies, they're likely to face challenges related to integration and scalability. Legacy systems, for example, may hinder the pace at which real-time processing can be employed. Ensuring robust data governance policies will be crucial in facilitating smooth transitions.
"The next wave of changes in big data will revolve around how quickly we can convert data into actionable insights."
Ethical Considerations in Big Data Business Models
In an era where data reigns supreme, the ethical dimensions of big data business models cannot be brushed aside. The interplay between ethics and data has far-reaching implications for both businesses and consumers. Understanding the ethical considerations helps companies not only protect themselves legally but also forge stronger relationships with their clients. Companies that prioritize ethical data practices are better positioned to gain trust and loyalty in a competitive environment.
Data Ownership and Control
When discussing data ownership, it's crucial to acknowledge that it’s not merely about who collects and stores the data, but also about who has the right to use it. In many cases, consumers share personal data without fully understanding the implications. This lack of awareness can lead to unintentional misuse of information. Companies must establish clear policies regarding data ownership, particularly in how they collect, use, and distribute this data.
Consider a scenario where a social media platform, like Facebook, utilizes user-generated content to enhance ad targeting. While the platform benefits from increased advertising revenue, users often feel alienated as they may not fully control their contributions. They find themselves in a gray area, where their data becomes a commodity, generating value for others while they face the risk of privacy breaches. Businesses should address this by implementing user-friendly consent processes and ensuring transparency.
The concept of control also extends to how companies respond to data breaches. For instance, if a healthcare provider suffers a data breach, patients want to know what steps are being taken to safeguard their information in the future. Organizations must not only protect data effectively but also communicate openly about their practices and policies surrounding data ownership.
Bias and Fairness in Analytics
Bias in big data analytics can lead to skewed results and unfair treatment of certain individuals or groups. Algorithms often reflect the prejudices of their creators or the data they are trained on. This raises concerns, especially when decisions about employment, loans, or healthcare are made based on potentially biased data. In recent years, instances of algorithmic bias have led to discrimination, with significant implications on some groups.
For example, facial recognition technologies used by various law enforcement agencies have faced criticism for disproportionately misidentifying people of certain racial demographics. This not only jeopardizes the integrity of security measures but also raises ethical questions about accountability in artificial intelligence developments.
To address bias, businesses should prioritize diversity in their data sources as well as in the teams developing these algorithms. Regular audits can help identify and mitigate biases, ensuring fairer outcomes in analytics. For a business operating in today's diverse environment, achieving fairness is not just good practice; it’s a critical aspect of brand reputation and trust.
In summary, ethical considerations in big data business models go beyond mere compliance. They influence brand integrity, consumer trust, and market position.
The discourse around ethical data practices should be ongoing, reflecting an evolving understanding of data’s role in society. By fostering transparency and fairness, organizations can not only adhere to ethical standards but also create a more inclusive digital landscape.
For further reading on the ethical implications of Big Data, you can explore resources from Wikipedia or Britannica.
Culmination and Implications for Investors
Big data business models represent a critical area for investors to grasp, as they navigate the burgeoning digital landscape. Understanding how data transforms industries is not just useful; it’s essential for making informed investment choices. With the rapid advancement of technology, the significance of data-driven insights cannot be overstated. The implications of these models stretch far beyond mere data collection and analysis; they influence market dynamics, consumer behavior, and even regulatory frameworks.
Emphasizing a few key elements helps clarify their importance:
- Value Creation: Big data isn’t just about volume; it’s about extracting actionable insights that drive profit. Investors need to recognize companies that can effectively leverage data to enhance decision-making processes.
- Risk Management: By analyzing trends, businesses can predict challenges and adapt accordingly. Investors should pay attention to how organizations utilize their data capabilities to mitigate risks and seize opportunities.
- Competitive Edge: Companies adept at big data analytics often outperform their rivals. This edge stems from the ability to innovate rapidly and pivot based on real-time information. Identifying such firms can lead to lucrative investment returns.
The benefits of thoroughly understanding big data business models are substantial. This knowledge helps investors differentiate between companies merely jumping on the data bandwagon and those embedding analytics deeply into their operations. The former may misallocate resources, while the latter could provide sustainable growth.
Furthermore, several considerations should also be on the radar:
- Investment Horizon: Are you in for the long haul, or looking for short-term plays? Big data initiatives often take time to yield significant returns.
- Sector Variability: Different sectors exhibit unique responses to big data’s potential. Investors must consider how industry-specific factors influence the effectiveness and applicability of data strategies.
A wise investor always seeks to connect the dots, understanding not just the numbers but the stories behind them. Big data models are those stories, revealing the narratives that can lead to smarter investments.
Key Takeaways from Big Data Business Models
- Data is an Asset: Treat it like one. Companies that can effectively nurture and monetize their data stand a better chance of succeeding.
- Evolution of Market Dynamics: Understanding how data reshapes markets is crucial for anticipating shifts.
- Innovation: Continuous development within big data technologies is imperative. Stay informed about emerging tools and techniques.
- Regulatory Compliance: Keeping abreast of legal and ethical considerations in data usage is vital for long-term sustainability.
Strategic Insights for Future Investments
- Diversify Investments in Data-Driven Companies: Don't put all your eggs in one basket. Spreading investments across different firms utilizing big data effectively can hedge against sector-specific risks.
- Focus on Partnerships: Companies that engage in strategic partnerships for data sharing and insights tend to amplify their success.
- Monitor Trends in AI and Machine Learning: As these technologies become more intertwined with big data, companies leveraging them will likely lead the pack.
- Assess Scalability: Look for businesses with scalable data platforms; smaller firms may struggle to grow as data demands increase.
In summary, the implications of understanding big data business models for investors are profound. By recognizing key takeaways and adopting strategic insights, investors can position themselves at the helm of innovation and benefit from a data-driven future.